Predictive vs. preventive
Published: 30 August, 2022
Both predictive and preventive maintenance are helpful to keep industrial equipment in good condition and to tackle problems before they occur. However, there is a big difference between the two. Neil Ballinger* offers an overview of the pros and cons of both methods.
With manufacturers constantly adjusting to cope with rising competition, rapidly changing consumer trends and pressure to deliver high-quality products quickly, equipment downtime can seriously compromise a business’ bottom lines.
According to a report published by maintenance specialist Senseye, manufacturers experience an average of 27 hours of downtime per month due to equipment failure, resulting in yearly revenue losses in the multi-millions.
Past vs present
Preventive — or preventative — and predictive maintenance are often used interchangeably in reference to maintenance strategies that allow manufacturers to act before equipment fails. Both methods are vastly superior to reactive maintenance, where equipment runs until emergency repairs are needed, often costing companies four to five times as much as proactive maintenance options, as reported by Operations & Maintenance Practices Guide, Release 3.0
However, preventive and predictive are not the same. Preventive maintenance involves checks at regular intervals, regardless of the equipment’s condition. It relies on best practice guidelines and historical data to give plant managers the best chances to keep machines in good repair but still requires cyclical planned downtime. However, the Operations & Maintenance Practices Guide also reports that predictive maintenance is estimated to save companies around 12 to 18% in costs compared to reactive maintenance.
Predictive maintenance, on the other hand, only occurs when needed, relying on real-time data from IIoT-connected equipment to identify potential threats before a problem occurs. In this way, repairs address an actual problem and are more targeted, meaning that downtime, when required, is reduced by 25 to 30% compared to other maintenance methods.
Storing big data
To work effectively, predictive maintenance relies on data from sensors that report on equipment’s health state. However, IBM estimates that about 90 per cent of all data generated by sensors goes unused. This means that manufacturers miss opportunities to make informed decisions about their equipment while still paying to collect and store data. Data collected but not processed or used in any way is known as dark data and represents a sizable challenge for the industry.
While sensors to collect data can be relatively inexpensive and easy to set up, the challenging part is processing the data to make conclusions on the health of the machine.
Processing data can be challenging for many reasons, from understanding the data to placing it with the relevant department. For example, data silos happen when data is processed and relevant patterns discovered, but these are not shared among the different departments of an organisation. This can happen due to the business not having the necessary technology for data visibility in place. For example, it might lack a unified integrated data management (IDM) tool or computerised maintenance management system (CMMS), and every team might rely on different platforms.
For example, current transformers can collect raw data on an electric motor’s eccentric rotors, winding issues, rotor bar issues and more. However, if there is no software such as CMMS to organise the data, this information might not be shared with the maintenance team on the production floor. This could result in unplanned downtime despite the use of sensors.
Lending a helping hand
Having sensors to gather information on equipment is the first step to incorporating the predictive technique into your maintenance strategy. Recent machines usually come with different options for realtime data acquisition, but legacy equipment can also be retrofitted with inexpensive addon sensors. In fact, predictive maintenance can be a vital asset when dealing with ageing equipment, which require careful planning when sourcing obsolete spare parts.
However, the impressive amount of dark data in the industry, coupled with the issue of data silos, shows that gathering data is not enough. To predict equipment failure effectively, manufacturers should implement technologies that facilitate real-time data processing and allow all relevant personnel to access the resulting insights.
To combat issues with sharing data among different departments, businesses should priorities the merging of information technology (IT) and operational technology (OT). The separate management of these teams due to different skill sets was once logical, but the increased digitalisation of manufacturing processes, including maintenance, means that it is now necessary to bring these areas together. OT collects raw data from PLCs, sensors and other key equipment, while IT gives the data meaning by uncovering relevant patterns. However, both equipment and teams must communicate and collaborate proactively.
One of the problems with data from industrial equipment is that the older it gets, the less relevant and accurate it becomes. In this sense, edge computing can be a valuable solution. By analysing data as close as possible to the source, rather than sending it all to the cloud, edge computing minimises latency and supports real-time decision making.
Edge computing can also assist with connecting more equipment to the IIoT — cybersecurity. When data travels back and forth from the cloud, there is an increased risk of it being compromised. Processing data closer to the source reduces this risk, offering the advantages of increased digitalisation without opening up more potential attack surfaces. This does not mean processing or storing data in the cloud should be avoided at all costs, but simply that the two options can go hand in hand to maximise results.
*Neil Ballinger is head of EMEA at automation parts supplier EU Automation
https://twitter.com/euautomation
https://www.linkedin.com/company/eu-automation/
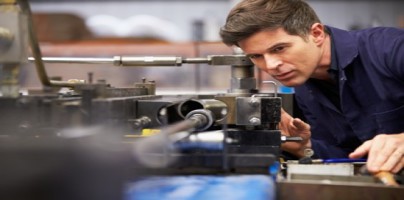